CPS for safe and sustainable aquaculture farming
This Application Experiment will substantially support sustainable aquaculture of safe and high quality products by digital control and self-learning automatization of biological processes involved.
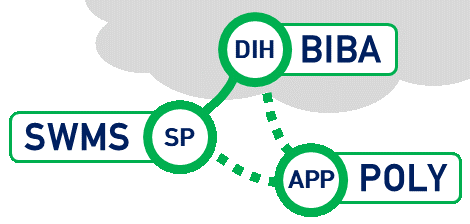
With this experiment, the participating companies want to meet challenges in the field of aquacultural farming using the opportunities given by digital technologies. Indoor aquaculture is a growing sector of food production and still in a young stage of development. The available processes for measuring and controlling such an aquaculture plant are largely based on traditional and manually executed production processes, measurements and calculations. Thus, many important parameters are not or only partially used for decisions that influence the process flow. Due to manual recording and processing, the parameters are only available at sporadic intervals, so that changes in the systems parameters do not result in a real time reaction.
All together this leads to significant inefficiencies in the areas of energy consumption, feed and medical supplies as well as poor water quality and fundamentally to poor performance of the overall plant.
Through the development of intelligent systems for data acquisition, data processing on the edge, data transfer to higher-level systems (on-premise or cloudbased), data analysis through machine learning and AI and the use of the information gained to generate control commands for the underlying processes it is highly likely that a more sustainable way of producing animal proteins/food is designed. With the project partners systems and algorithms will be developed and tested in test setups as well as in a real production environment in an aquacultural plant for white tiger shrimps.
Partner | Expertise | Role |
---|---|---|
POLY | Engineering and development of solutions for water treatment and indoor aquaculture plants | Application engineering, field trials on test-farm for evaluation of developed technological solutions |
SWMS | Experts for digitalization of industrial processes using IoT and IT technologies | Enhancement of IoT technology to food processing and biological monitoring. Development of ML/AI methods for biological processes |
BIBA | Research institute for production & logistics. CPS/IoT test fields. | Enhancement of embedded systems with camera technologies. Integration of image recognition/AI/ML methods on the edge in cooperation with SWMS. |
Problem description
To date operators need to estimate the live biomass, based on assumptions on growth and survival rate, in order to have a guess on how much feed the organisms cultured will need each day. This leads to underfeeding or overfeeding, both of which are related to negative effects: either reduced growth or reduced water quality, in turn creating reduced growth. A sustainable production requires the knowledge of how much live organisms are present at any time, giving reliable values for their feed demand. The relevant parameters are:
Online Definition of live biomass and biofloc concentration:
- Shrimp biomass [kg/m³], mean sizes [cm] and body weights [g/pcs]
- Biofloc concentration in grow out tanks (l/m³) and mean size of flocs [µm]
The daily feed input directly relates to the live biomass in a system. Both overfeeding and underfeeding has negative impacts on the production. Knowing this value allows for more accurate feeding, optimizing the growth, feed conversion and the water quality. The concentration of bioflocs has to be monitored daily, so far manually, since the water treatment has to be operated to maintain a stable level of newly developing bioflocs, to control their function of nitrification and their nutritive value. Aged and nutrient-deficient bioflocs need to be removed from the system. This process can be automatized as well.
Biofloc concentration in water treatment (bioreactor) (l/m³) and mean size of flocs [µm]
Online definition of the concentration of dissolved NO3-N and NO2-N [mg/l] in all compartments
Nitrite is toxic to fish and shrimp and to be controlled processes that nitrification to Nitrate. Nitrate is far less toxic, but will accumulate in systems without water renewal, such as biofloc systems. Therefore, these systems need a denitrification step in the water treatment, and this process can be enhanced and operated most efficiently by knowing the exact nitrate levels.
More parameters could be:
- Concentration of Oxygene in nursery and grow-out tanks
- pH value in grow-out tanks and water treatment
- oxidation-reduction potential ORP) [mV] in all compartments
- Temperature values (water & air)
A decrease in oxygen, pH and ORP is an indicator for overfeeding (uneaten feed decreases water quality).
This process automation can only be achieved by on-site technology and this technology needs to be integrated in intelligent automatization systems, connected to other important parameters (e.g. water & biomass quality) and all operational processes, in order to achieve full automation, the best results and a truly sustainably produced product of high and safe quality. Monitoring the biomass at real-time and connecting this core-value with operations, such as aeration, feeding, water circulation etc.
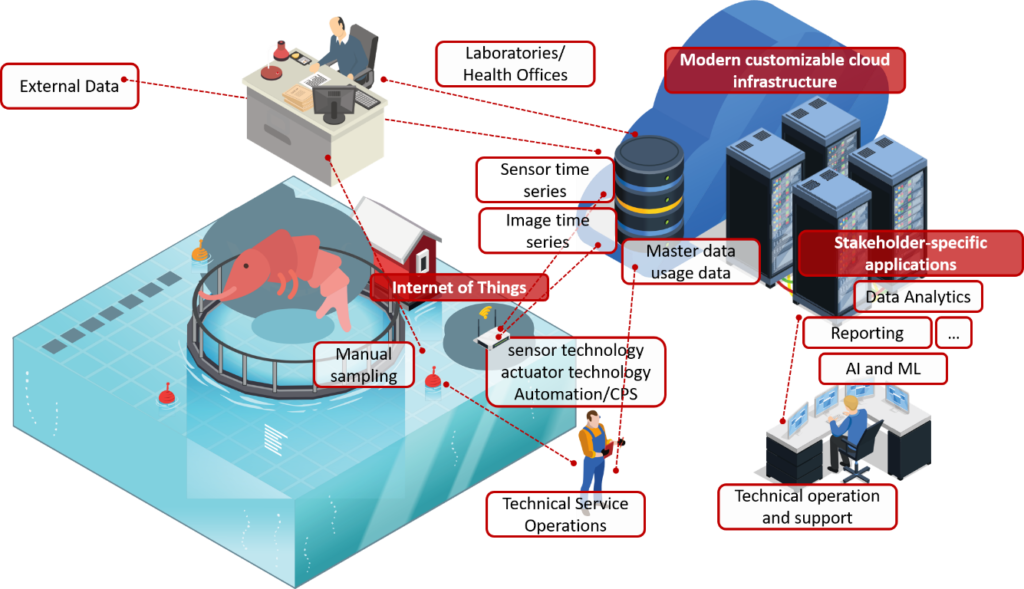
In order to achieve this, the project partners are developing a digital twin of the aquaculture production plant. The following steps are necessary:
Data acquisition: The process chain will be equipped with sensors in order to obtain the required data. This sensor technology has already partly been developed already and can be purchased (e.g. temperature and pH value). On the other hand it is necessary to develop own sensor technology. For this purpose, the use of techniques from the field of image processing is planned. With this technology, for example, the existing biomass and the size of mediums in the plant can be monitored in the production process.
Data processing: The data obtained is processed on an embedded system using machine learning algorithms and AI algorithms, which have to be developed. E.g. neural networks can be used to determine the various relevant parameters. At the same time, it is necessary to conduct research into the required database structure, according to the requirements of asset, process, product and biology tracking
Development of feedback control approaches: In a final step, the aim is to fully automate the process of producing animal proteins. The system independently controls various process parameters with the aim of minimizing or maximizing previously defined target values.
Business aspects: As described, the manufacturing process in the field of aquaculture is underdeveloped with respect to digitalization. Society is looking for sustainable ways of producing food in order to meet the growing food demand and at the same time reduce environmental pollution. To this purpose, aquacultures are one possible solution as they can provide high quality food. From the company’s point of view, on top of that, reducing process costs and increasing product quality has a double effect on a company with the aim of earning money.
Approach
The project partners follow the approach of combining their experiences in the fields of sensor technology, data processing, digitalization and the operation of aquaculture plants in order to meet the already described possibilities of process and quality improvements. In the field of food production the aim is to develop a digital twin by building cyber physical systems. In addition to improving the sustainability of food production, care should also be taken in all project steps to ensure that the solutions developed not only run in a test environment, but can also be applied to the real world. This includes not only the circumstances from the plant operations, but also the aspect of economic feasibility.
Implementation plan
- Comprehensive requirements engineering to describe the interests of all stakeholders and to identify the most decisive process steps.
- Preparation of a business plan
- Development of a detailed project plan and distribution of tasks between the project partners.
- Construction of a prototype for the acquisition of image data
- Development of a prototype for the processing the recorded data
- Use of the information gained for the development of process control algorithms
- Combination and optimization of prototypes into one system
- Further development of the system for productive use (TRL 7)
Expected results
It is expected that it will be possible to monitor the complete production process by using a digital twin concept by means of embedded systems and cyber-physical systems. This will increase safety and quality in food production and minimise waste of energy and pet food. This results in research and innovation enhances application and state of practice in the areas of ML, AI and image processing, in particular in a so far under-developed application domain. At this, a prototype is developed which will be used in a productive environment and which uses AI/ML methods to control and improve the processes themselves.